AI for Researchers: The Ultimate Guide to Supercharging Your Scientific Discovery
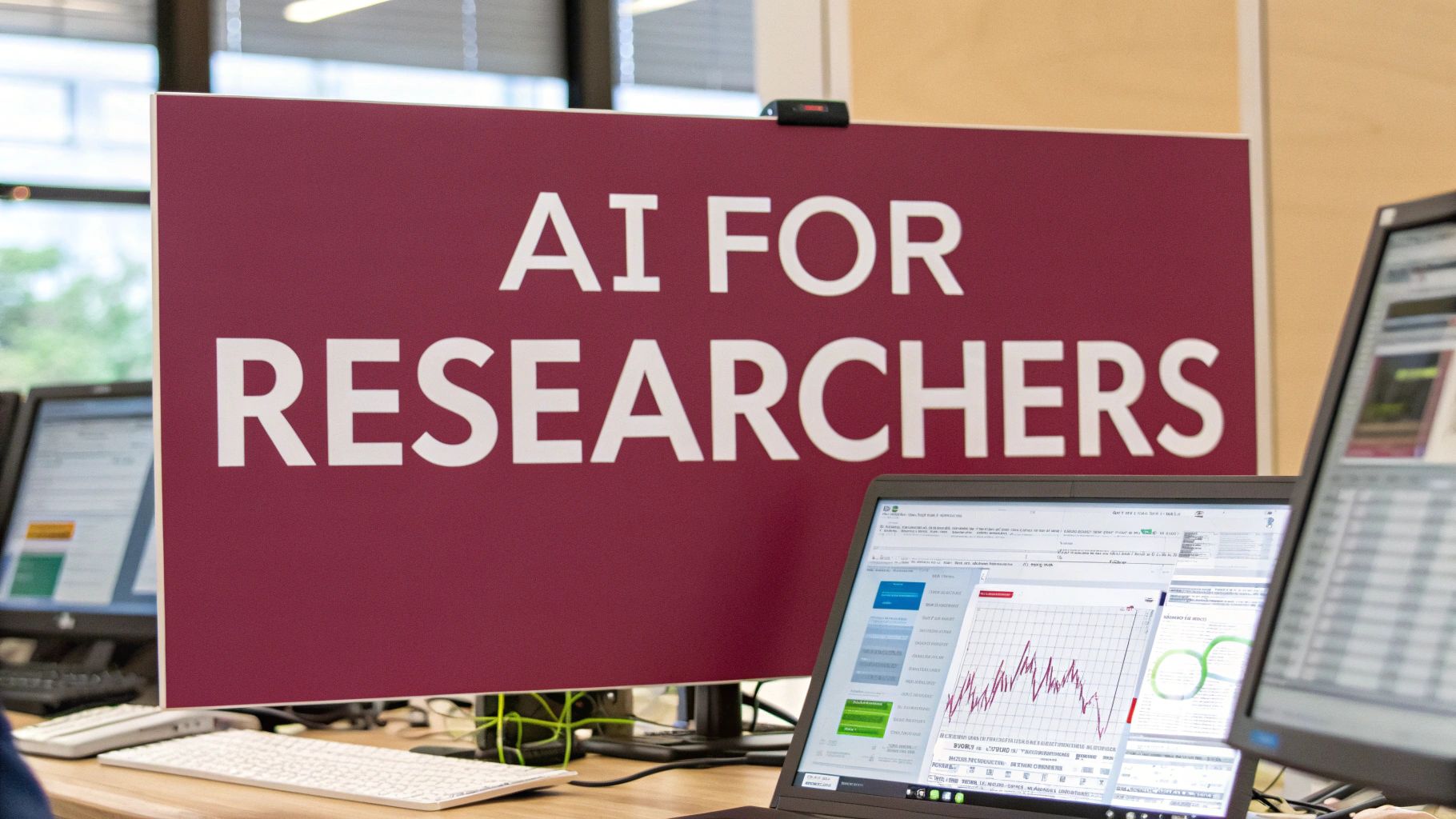
Breaking Through Research Barriers with AI

Researchers today face both exciting possibilities and real challenges when incorporating AI into their work. Success requires a thoughtful, systematic approach to implementation. One key challenge is sorting through the many available AI tools to find the right ones. For instance, scientists studying protein folding must evaluate multiple machine learning algorithms designed for this specific purpose. This makes tool selection a critical first step.
Choosing the Right AI Tools for Your Research
Just as researchers carefully select traditional tools for their work - using microscopes for cell studies rather than telescopes - they must thoughtfully match AI tools to their specific research needs. The tool's ease of use and compatibility with existing research processes are essential considerations. Even a powerful AI system can slow research down if it requires extensive training or specialized setups. This makes understanding each tool's core functions vital.
Understanding the Core Capabilities of AI Tools
Research-focused AI tools span a wide range - from data analysis algorithms to literature review assistants powered by natural language processing. Some excel at finding patterns in large datasets, while others specialize in making predictions or summarizing text. However, no single tool works perfectly for every research scenario. Each has specific strengths and limitations that researchers must weigh carefully before adding them to their workflow.
Overcoming Common Implementation Hurdles
Once tools are selected, practical challenges often emerge during implementation. Data preparation, while essential, can consume significant time. AI systems need clean, properly formatted data to function well. This often means researchers must invest considerable effort in organizing and preprocessing their data before they can use AI tools effectively. Another key challenge is making sense of AI-generated results. While AI can spot patterns and make predictions, researchers need ways to understand and validate the reasoning behind these outputs to ensure scientific rigor.
Balancing AI Capabilities with Human Insight
The most effective research approach combines AI's analytical power with human expertise. While AI tools can process vast datasets and identify hidden patterns efficiently, human researchers remain essential for developing research questions, interpreting findings, and addressing ethical considerations. The key is viewing AI as a powerful research assistant that extends human capabilities rather than replaces them. This collaborative relationship between researchers and AI tools will continue shaping how we advance scientific knowledge.
Mastering AI-Powered Literature Review
Researchers face an ongoing challenge - processing the massive volume of academic papers published daily. Finding relevant information among thousands of studies requires significant time and effort. Fortunately, AI tools now offer researchers powerful ways to efficiently analyze scholarly works while maintaining academic rigor. By combining advanced AI capabilities with human expertise, researchers can conduct more comprehensive and insightful literature reviews.
Smart Paper Selection and Efficient Data Extraction
AI excels at identifying relevant research papers through semantic analysis rather than just keyword matching. For example, when researching environmental impacts on agriculture, AI can surface valuable papers about climate change effects on crop yields, even if they don't use the exact search terms. This semantic understanding helps researchers discover important related work they might otherwise miss. AI tools can also automatically extract key details like methodologies and results from multiple papers, dramatically reducing manual data collection time.
Synthesizing Findings and Identifying New Connections
AI helps researchers see the bigger picture by analyzing patterns and relationships across many studies. Rather than just summarizing individual papers, AI can reveal emerging trends and potential conflicts in the literature. This broad perspective often highlights unexpected connections between different fields - for instance, showing how findings from materials science could advance biomedical engineering applications. These cross-disciplinary insights spark new research directions that may not be obvious through traditional review methods.
Combining AI-Powered Tools With Human Expertise
While AI accelerates literature reviews, human judgment remains essential. Researchers must carefully evaluate AI-identified papers and extracted data for relevance and accuracy, especially given potential algorithmic biases. The researcher's expertise is crucial for interpreting synthesized findings and developing new research questions based on AI-generated insights. Think of AI as a highly capable research assistant - it excels at gathering and organizing information, freeing researchers to focus on analysis and critical thinking. This partnership between AI tools and human expertise leads to more efficient and comprehensive literature reviews while maintaining scientific rigor. The goal is to enhance, not replace, human researchers' ability to advance scientific knowledge through thoughtful analysis and interpretation.
Designing Smarter Experiments with AI

AI tools are reshaping how researchers plan and conduct experiments. By analyzing large datasets and spotting key patterns, AI helps scientists develop more precise and effective experimental designs. The technology offers practical advantages - from optimizing study parameters to identifying hidden variables that could affect results.
Optimizing Study Design and Parameter Selection
AI excels at finding the best parameters for experimental design by analyzing vast datasets. For example, in pharmaceutical research, AI systems can evaluate thousands of chemical compounds to determine which ones show the most promise against specific diseases. This focused approach helps researchers test only the most viable candidates. Similarly, when planning clinical trials, AI assists in determining optimal dosages, treatment durations, and control group sizes to produce statistically meaningful results.
Predicting Experimental Outcomes and Reducing Uncertainty
Before conducting actual experiments, AI can help forecast likely outcomes by finding patterns in historical data that humans might miss. For instance, materials scientists use AI to predict how new compounds will behave based on their composition and structure. This allows them to focus their lab work on the most promising materials rather than testing every possibility. The result is fewer wasted resources on experiments unlikely to succeed.
Identifying Hidden Variables and Mitigating Bias
One key strength of AI in research design is spotting hidden factors that could skew experimental results. These confounding variables often lead to incorrect conclusions when not properly controlled. AI analysis helps identify potential sources of bias so researchers can account for them in their study design. In social science research, for example, AI tools can point out demographic factors that might influence survey responses, allowing researchers to adjust their methods accordingly.
Generating Novel Hypotheses and Exploring New Research Directions
Beyond refining existing research approaches, AI opens up new possibilities by uncovering unexpected connections in data. This ability to spot novel patterns helps researchers develop fresh hypotheses they might not have considered otherwise. Scientists can then explore these AI-generated research questions, leading to discoveries in unexplored areas. The partnership between human researchers and AI systems creates opportunities to advance scientific knowledge in ways neither could achieve alone.
Building AI-Enhanced Research Collaborations
Research teams are discovering new ways to work together effectively using AI tools. These technologies help teams share data, coordinate projects, and communicate across disciplines and locations. Let's explore how AI is making research collaborations more productive and impactful.
Streamlining Data Sharing and Management
AI makes data sharing simpler by helping researchers organize and tag large datasets efficiently. Consider a team of biologists studying climate change effects on plants across multiple continents. They use AI tools to standardize data formats, automatically add metadata, and control access permissions - allowing team members to find and use data regardless of location. This means less time spent organizing data and more time analyzing it. AI platforms also help connect researchers with relevant datasets from other institutions. This broader access to data often leads to stronger research findings and new insights.
Enhancing Project Coordination and Communication
AI project management tools help research teams stay organized and work together smoothly. These tools track task progress, manage schedules, and keep communication flowing between team members. For example, AI can create meeting summaries, monitor document changes, and find meeting times that work for everyone. This reduces administrative work and keeps projects moving forward. AI also helps bridge communication gaps between researchers from different fields by explaining technical terms and highlighting related work. This leads to better understanding between disciplines and more creative problem-solving.
Fostering Interdisciplinary Collaboration and Knowledge Exchange
AI makes it easier for researchers from different fields to work together by improving communication and data sharing. Picture a project with computer scientists, doctors, and biologists developing new medical diagnostic tools. AI helps translate complex terms, find common research interests, and combine different types of data. When experts from various fields can easily share ideas and work together, they often develop solutions that wouldn't be possible otherwise. AI platforms also help researchers find potential collaborators with similar interests and expertise, creating new research networks worldwide. This expanded collaboration gives researchers access to more diverse perspectives and resources, leading to faster scientific progress and discovery.
Navigating AI Ethics in Research

As research increasingly incorporates artificial intelligence tools, new ethical questions arise that go beyond technical considerations. While AI enables exciting research possibilities through collaboration and data sharing, researchers must carefully consider how to use these tools responsibly and ethically.
Addressing Bias and Ensuring Fairness in AI Research
When AI models learn from historical data, they can absorb and amplify existing societal biases. For instance, if an AI analyzes past research grant data where certain groups received less funding, it may continue those patterns of inequity. To prevent this, researchers need concrete steps to identify and reduce bias. These include expanding training data to be more representative, adding fairness constraints to algorithms, and testing extensively across different populations. Being open about data sources and how algorithms work allows others to check for potential bias issues.
Maintaining Transparency and Explainability in AI-Driven Research
Many AI models operate as "black boxes," making decisions in ways that are hard to understand. This lack of transparency can reduce trust in research findings. That's why researchers should focus on explainable AI approaches that make the decision-making process clearer. For example, creating visualizations that show which factors most influenced an AI's conclusions helps validate the results. Detailed documentation of AI methods also lets other researchers examine and build upon the work.
Protecting Sensitive Data in the Age of AI
Research with AI often involves private information like medical records or personal details. This requires strong security measures to prevent data breaches or misuse. Key practices include removing identifying information, using secure storage systems, and carefully controlling who can access the data. Researchers must also follow relevant privacy regulations and get proper consent from people whose data they use, especially in fields like healthcare research.
AI Attribution and Building Trust
Just as researchers cite human collaborators, they need to properly credit AI tools used in their work. This means clearly explaining which AI systems they used, what those tools did, and how they shaped the research process and findings. Being upfront about both the capabilities and limitations of AI helps build credibility with other scientists and the public. This open approach allows the research community to work together on improving ethical AI practices. By actively addressing these ethical considerations, researchers can ensure AI remains a valuable tool that advances knowledge responsibly.
Preparing for Tomorrow's Research Landscape

The research landscape is changing rapidly as AI tools become increasingly central to scientific work. To stay competitive, researchers must not only master current AI technologies but also develop skills to evaluate and adopt emerging ones. This shift is happening at an unprecedented pace - for example, generative AI adoption has outpaced even the early adoption rates of revolutionary technologies like personal computers and the internet.
Identifying Promising AI Research Tools and Methodologies
Staying current with AI developments requires an active approach to learning and assessment. Researchers should regularly read new publications, attend relevant conferences, and engage with AI developers and research leaders in their field. Understanding AI development trends helps identify which new technologies deserve focused attention. For instance, the surge in funding and new companies focused on generative AI points to its growing importance in research.
Evaluating and Adapting to New AI Tools
Just as researchers carefully assess traditional research methods, they need clear criteria for evaluating AI tools. Key considerations include accuracy, efficiency, ease of use, and workflow integration. Beyond technical specifications, researchers should examine how new AI tools might reshape their research process. For example, a new AI analysis tool might enable different experimental approaches or data collection methods. Taking time to plan these adjustments helps ensure smooth integration of AI tools into existing research practices.
Positioning Your Research for Future Breakthroughs With AI
AI offers more than just efficiency gains - it opens up entirely new research possibilities. For example, AI can analyze massive datasets to reveal hidden patterns that spark novel hypotheses, or it can identify unexpected connections between seemingly unrelated research areas. By thoughtfully applying AI capabilities, researchers can explore questions that were previously impractical or impossible to investigate. This combination of human insight and AI analysis capabilities creates opportunities for major scientific advances.
Preparing Your Research Team for the Future of AI
As AI becomes fundamental to research, teams need proper training and support to use these tools effectively. This includes teaching technical skills for working with AI systems as well as developing good judgment about interpreting AI-generated results. Teams should openly discuss ethical considerations around AI use in research. Since AI capabilities evolve quickly, creating a culture of continuous learning helps research groups adapt and thrive with new developments.
Ready to experience the power of AI in your research? AnswerThis, an AI-powered research assistant, helps you efficiently access and understand information by generating comprehensive literature reviews with line-by-line citations. Visit https://answerthis.io/ to learn more and start supercharging your research today.